CSM-Toxin predicting the toxicity of biologics
Vladimir Morozov, Carlos H.M. Rodrigues & David B. Ascher
Abstract: Biologics are one of the most rapidly expanding classes of therapeutics, but can be associated with a range of toxic properties. In small molecule drug development, early identification of potential toxicity led to a significant reduction in clinical trial failures, however we currently lack robust qualitative rules or predictive tools for peptide and protein based biologics. To address this, we have manually curated the largest set of high quality experimental data on peptide and protein toxicities, and developed CSM-Toxin, a novel in-silico protein toxicity classifier, which relies solely on the protein primary sequence. Our approach encodes protein sequence information using a deep learning natural languages model to understand the “biological” language, where residues are treated as words and protein sequences as sentences. CSM-Toxin was able to accurately identify peptides and proteins with potential toxicity, achieving a MCC up to 0.66 across both cross-validation and multiple non-redundant blind tests, outperforming other methods and highlighting the robust and generalisable performance of our model. We strongly believe CSM-Toxin will serve as a valuable platform to minimise potential toxicity in the biologic development pipeline.
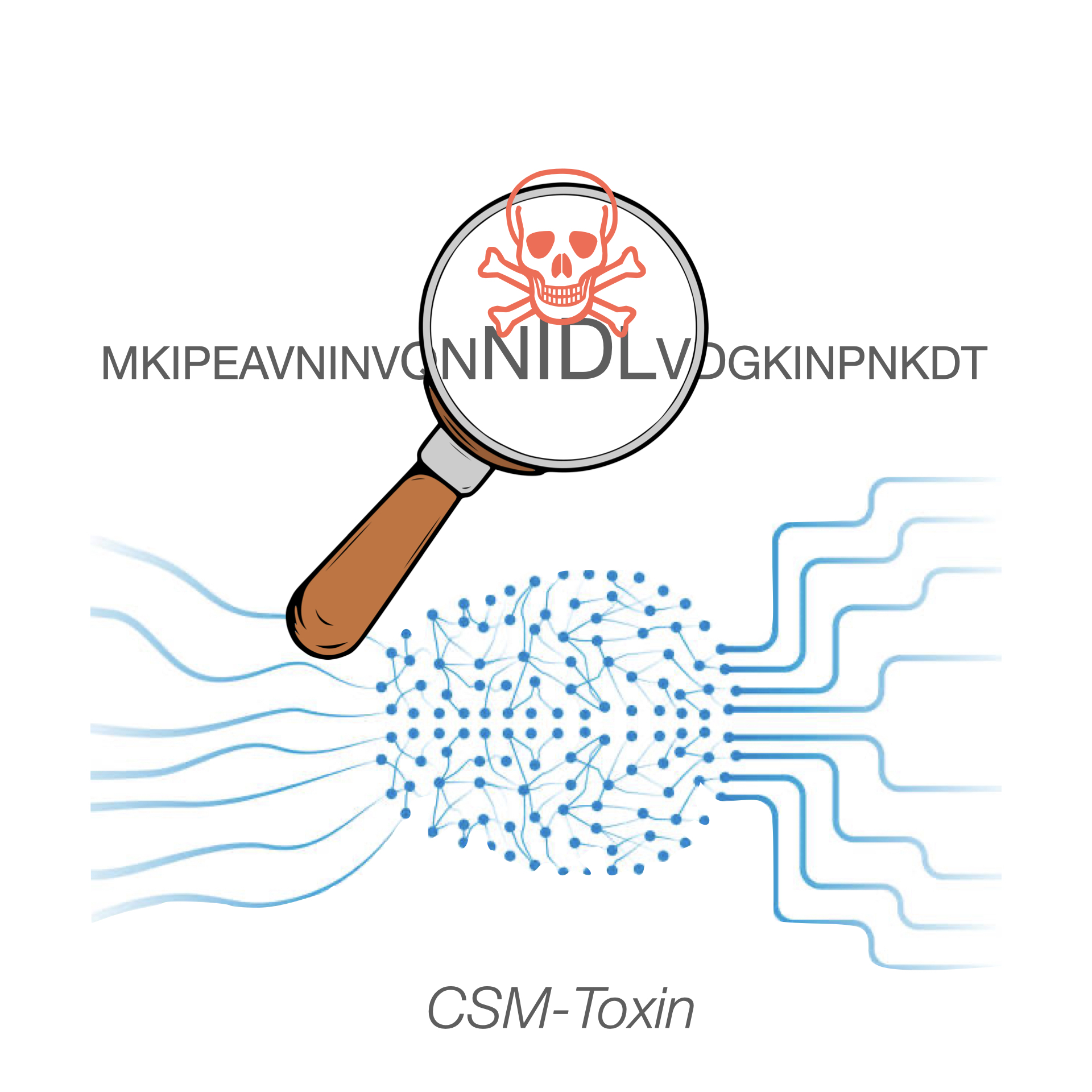