EFG-CS Predicting Chemical Shifts from Amino Acid Sequences with Protein Structure Prediction Using Machine Learning and Deep Learning Models
Dawn Gu, Yoochan Myung, Carlos H.M. Rodrigues, David B. Ascher
Abstract: Nuclear magnetic resonance (NMR) crystallography is one of the main methods in structural biology for analysing protein stereochemistry and structure. The chemical shift of the resonance frequency reflects the effect of the protons in a molecule producing distinct NMR signals in different chemical environments. Apprehending chemical shifts from NMR signals can be challenging since having an NMR structure does not necessarily provide all the required chemical shift information, making predictive models essential for accurately deducing chemical shifts, either from protein structures or, more ideally, directly from amino acid sequences. Here, we present EFG-CS, a web server that specialising in chemical shift prediction. EFG-CS employs a machine learning-based transfer prediction model for backbone atom chemical shift prediction, using ESMFold-predicted protein structures. Additionally, ESG-CS incorporates a Graph Neural Network-based model to provide comprehensive side-chain atom chemical shift predictions. Our method demonstrated reliable performance in backbone atom prediction, achieving comparable accuracy levels with root mean square errors (RMSE) of 0.30 ppm for H, 0.22 ppm for Ha, 0.89 ppm for C, 0.89 ppm for Ca, 0.84 ppm for Cb, and 1.69 ppm for N. Moreover, our approach also showed predictive capabilities in side-chain atom chemical shift prediction achieving RMSE values of 0.71 ppm for Hb, 0.74 to 1.15 ppm for Hd, and 0.58 to 0.94 ppm for Hg, solely utilising amino acid sequences without homology or feature curation.
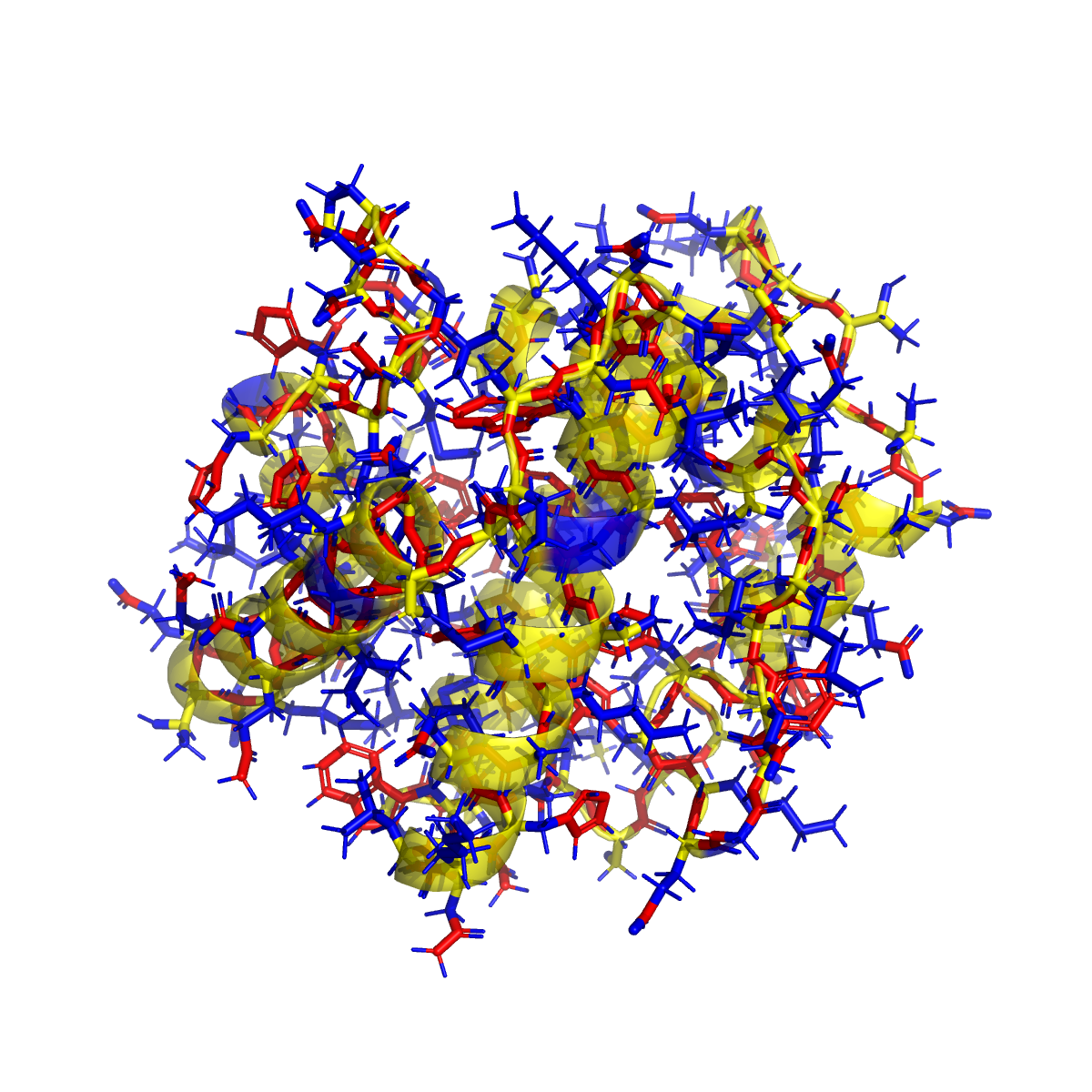